A significant concern with the rise of artificial intelligence is its potential to replace human jobs. When it comes to the work of nutritionists and dietitians, large language models like ChatGPT are prime candidates for such replacement.
Introduction
Nutrition scientists worldwide broadly agree on what constitutes a healthy diet. That’s why most countries’ food-based dietary guidelines emphasise a similar dietary pattern: one rich in fruits, vegetables, whole grains, nuts, seeds, plant-based proteins (such as beans and lentils), lean animal proteins (like fish and poultry), and moderate amounts of dairy or fortified plant-based alternatives (1).
However, you’ll be forgiven for thinking that there is serious confusion about what constitutes a healthy diet if your trusted source of information is social media headlines and influencers. In a recent systematic review of nutrition-related information on websites and social media, about 50% of information was reported to be inaccurate or of low quality (2). Because diet plays an important role in overall health, dietary dis-/misinformation can cause serious harm.
Therefore, it would be beneficial for the public to have access to technology that can help when it comes to making healthy dietary choices. Which is where we come to large language models like ChatGPT—forms of artificial intelligence (AI) that can understand human text and provide informed answers to various questions. Wouldn’t it be great if we could receive accurate, evidence-based dietary information from this technology? I think so, although one could argue that this could spell danger for the profession of nutrition and dietetics.
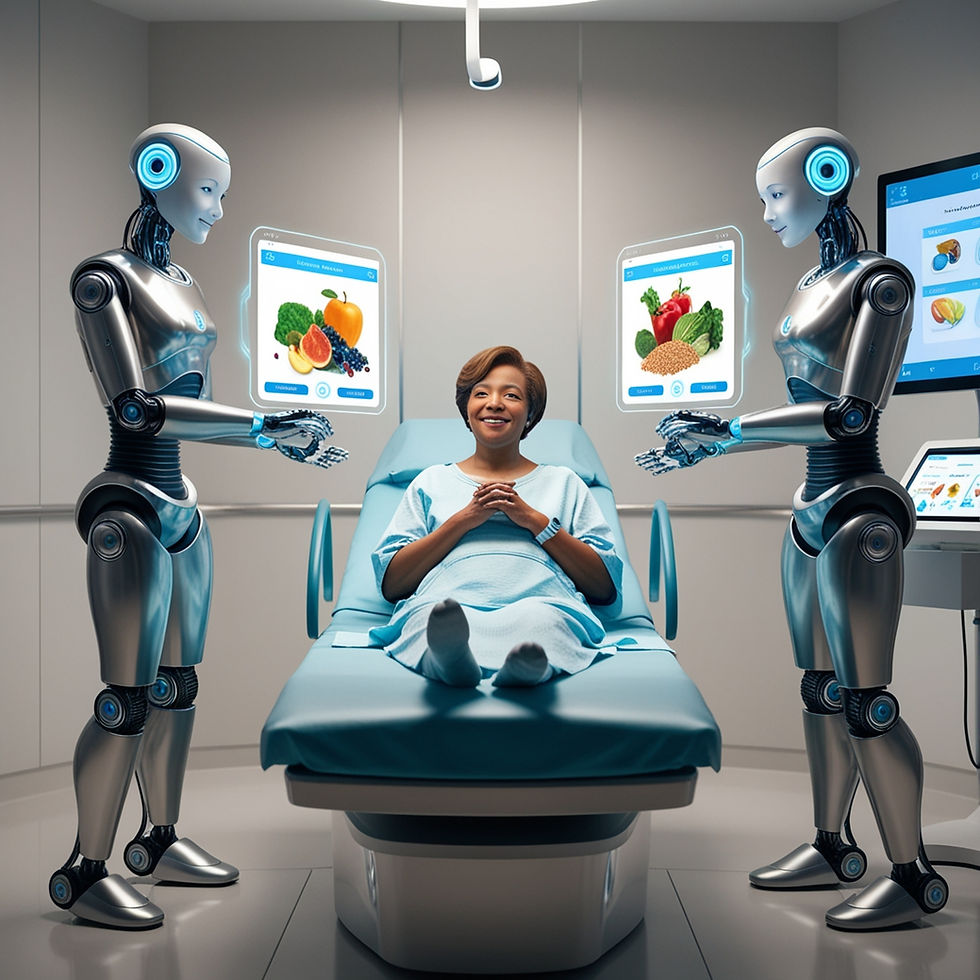
Large Language Models
Large language models like OpenAI’s ChatGPT or Google’s Gemini are trained on large datasets that include books, articles, and websites (3). This extensive training allows them to retrieve and convey information on a wide range of topics. If asked questions on specific topics such as nutrition, large language models will provide coherent personalised responses using the most probable and relevant words. These answers can be generated 24/7 and can range from very detailed to very basic in nature, depending on the prompt.
However, it is important to note the limitations of large language models. First of all, their knowledge is often dated, meaning that they may not be aware of new discoveries or research related to a specific topic, which could impact the accuracy of their responses. In addition, they are not perfect; they make mistakes. In fact, I chronicled some mistakes from ChatGPT to my own nutrition-related questions in a previous Instagram post. Therefore, at least from my experience, I would advise to take their answers with a pinch of salt. Thankfully, we don’t have to rely on my personal experience because a handful of studies have been conducted to assess the ability of large language models to generate evidence-based nutrition information.
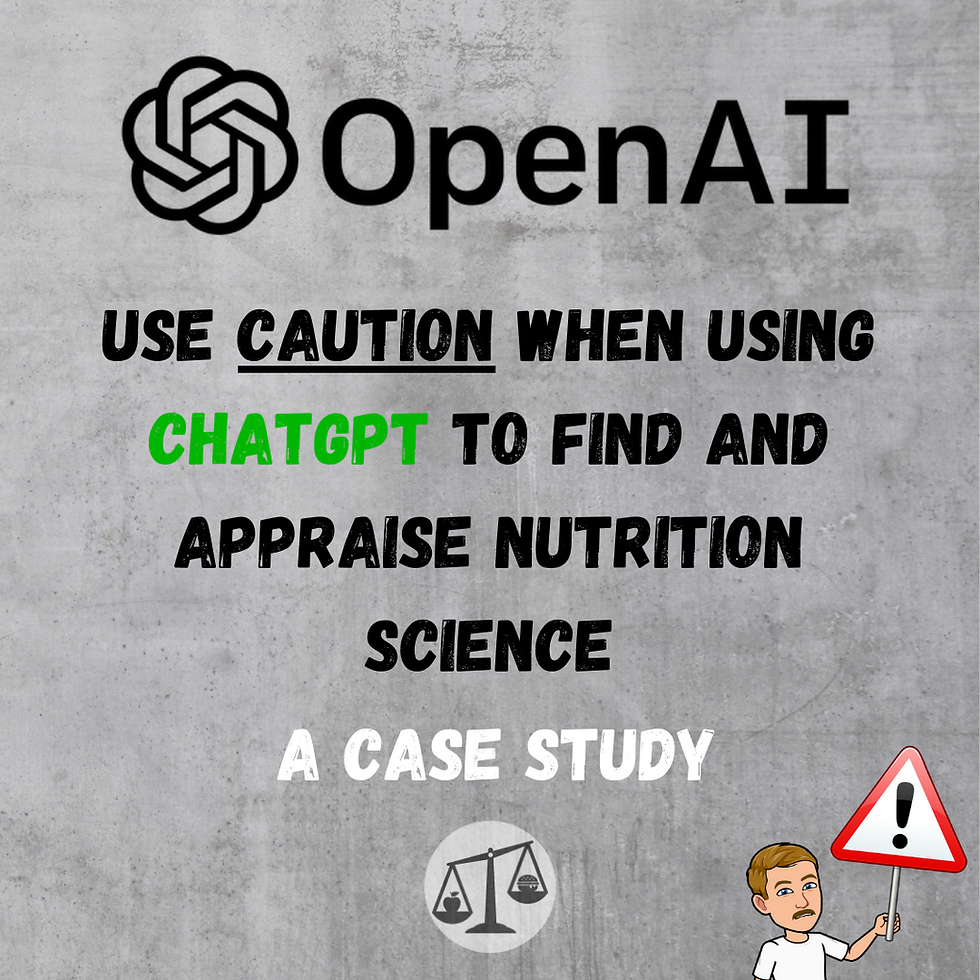
My Instagram post detailing inaccuracies from ChatGPT.
Large Language Models as Nutritionists
In a study where answers to common questions received in dietetic practice were provided separately by 7 dietitians and ChatGPT, the answers from ChatGPT were more often deemed to be of higher quality (in terms of ‘scientific correctness’, ‘actionability’, and ‘comprehensibility’) by a panel of 27 nutrition experts (4). In another study, where answers to nutrition-related questions from ChatGPT were assessed by 30 dietitians, the answers were often deemed to be readable, but lacked understandability, practicality, and completeness (5).
In a study where ChatGPT was tasked with coming up with nutritional guidance for specific chronic diseases, its answers were deemed to be appropriate ~55–73% of the time by 2 nutrition experts (6). In addition, very few recommendations were deemed to be inappropriate, but ChatGPT did produce contradictory recommendations when it was tasked with providing nutritional guidance for a hypothetical patient with multiple chronic diseases (6).
In a study where ChatGPT was tasked with coming up with meal plans for a hypothetical 30-year old woman with various food allergies, almost all meal plans generated were free of the allergen of interest except for the ‘nut-free’ meal plan, which included almond milk (7). The researchers also asked ChatGPT to come up with allergen-free meal plans specific to certain energy requirements (i.e., 2,000 calorie and 1,000 calorie diets), which it struggled to do accurately (7).
In another study investigating ChatGPT’s ability to develop meal plans for various dietary patterns (i.e., omnivorous, vegetarian, and vegan), results showed that meal plans were on average low in carbohydrate and energy, low in vitamin B12 for vegan meal plans, low in iron for omnivorous meal plans, and consistently low in fluoride and vitamin D across meal plans, relative to recommendations (8). This study was the only one to compare ChatGPT to Gemini, and seemed to show a slight advantage to ChatGPT (8). This is because Gemini sometimes included foods not compatible with the dietary pattern (e.g., milk and eggs in a vegan diet), whereas ChatGPT didn’t, and Gemini never recommended vitamin B12 in any vegan dietary plan, whereas ChatGPT did so in 5/18 cases (if you did not know, vitamin B12 must be taken as a supplement or eaten through fortified foods if eating a vegan diet, as it is not naturally found in plant foods) (8).
In another study that evaluated ChatGPT’s ability to estimate energy and macronutrient content of 8 menus designed for adults, there were no significant differences between ChatGPT and nutritionist estimations of energy, carbohydrate, and fat content of menus; however, ChatGPT overestimated protein composition (9). This study was the only one to compare ChatGPT-3.5—the free version—to ChatGPT-4—the paid version—finding that ChatGPT-4 more closely matched nutritionist estimations (except for protein, which it overestimated to a greater extent than ChatGPT-3.5) (9).
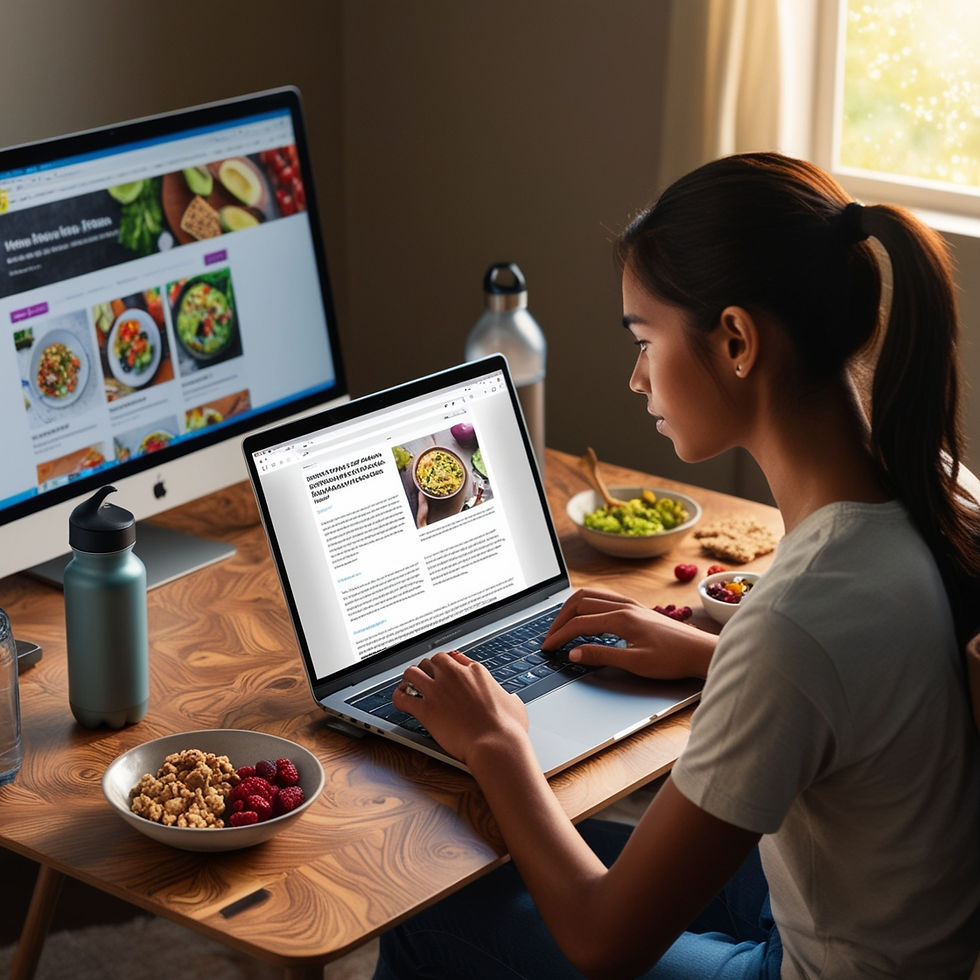
Main Takeaways
In this growing area of research, the findings are, in my opinion, quite promising. In particular, it seems that ChatGPT is pretty good at providing broad dietary recommendations for specific nutrition-related questions, as well as for the management of chronic diseases. Where large language models seem to struggle is in the estimation of energy and nutrient content of meals and dishes. However, as a tool for understanding how to eat healthier, or to better understand certain nutrition-related questions, I would be quite positive about ChatGPT at least.
To circle back to the title of this article: no, ChatGPT cannot replace nutritionists in its current format. However, I do believe that it has a lot of potential to provide individuals with instant, evidence-based dietary advice at a fraction of the cost of visiting a nutritionist or dietitian. In fact, I think that nutritionists and dietitians could make effective use of this technology in their practice, perhaps using it to answer nutrition-related questions and queries to allow more time to develop behaviour change strategies for their clients (4). In addition, ChatGPT can be used in conjunction with more sophisticated forms of AI to create personalised meal plans (10).
Despite my optimism, it’s clear that there are some issues with large language models. For example, ChatGPT recommended almond milk in a nut-free diet plan, which could be deadly for someone with a severe nut allergy. Further, in the study that investigated ChatGPT’s ability to develop meal plans for various dietary patterns, responses varied by 20–30% depending on the account used to ask questions of ChatGPT and Gemini (8). This suggests that large language models may not be reliable in the information they provide to different accounts (or people).
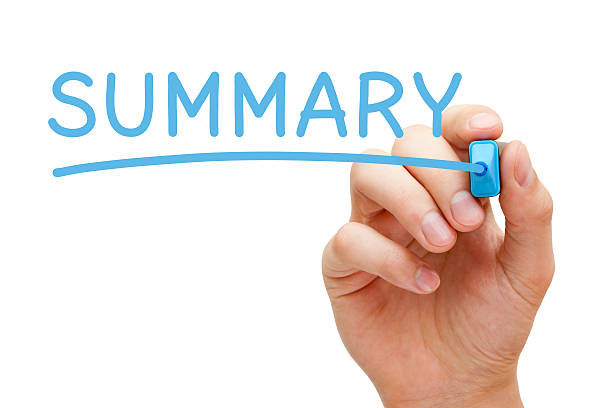
Summary
All in all, I’m quite optimistic about the potential of ChatGPT to provide evidence-based nutrition advice. However, I would still like to stress that this technology will make mistakes, so continue to take the information generated with a pinch of salt. In addition, one issue not often discussed about using large language models is their considerable environmental impact—driven by the energy-guzzling data centres that are required by AI. So if more and more people are using this technology to receive nutrition advice, this would be less than ideal from an environmental point of view and is something to keep in mind. Ultimately, I would still recommend booking in with a registered dietitian or nutritionist for evidence-based nutrition guidance.
If you are interested in levelling up your football skills through supplemental football training, contact us at admin@training121.com to book in with our coaches for a session. And remember to sign up to our (spam-free) mailing list to be notified when a new blog article drops.
Thanks for reading!
Patrick Elliott, BSc, MPH
Health and Nutrition Science Communication Officer at Training121
Instagram: @just.health.info
Twitter/X: @PatrickElliott0
References
(1) Cámara M, Giner RM, González-Fandos E, López-García E, Mañes J, Portillo MP, Rafecas M, Domínguez L, Martínez JA. Food-Based Dietary Guidelines around the World: A Comparative Analysis to Update AESAN Scientific Committee Dietary Recommendations. Nutrients. 2021;13(9):3131. Available at: https://www.mdpi.com/2072-6643/13/9/3131
(2) Denniss E, Lindberg R, McNaughton SA. Quality and accuracy of online nutrition-related information: a systematic review of content analysis studies. Public Health Nutr. 2023;26(7):1345–57. Available at: https://www.cambridge.org/core/journals/public-health-nutrition/article/quality-and-accuracy-of-online-nutritionrelated-information-a-systematic-review-of-content-analysis-studies/4E28F7A056AA8CB4A19F9E5DF0B095C2
(3) Arslan S. Decoding dietary myths: The role of ChatGPT in modern nutrition. Clin Nutr ESPEN. 2024;60:285–8. Available at: https://clinicalnutritionespen.com/article/S2405-4577(24)00047-0/abstract
(4) Kirk D, van Eijnatten E, Camps G. Comparison of Answers between ChatGPT and Human Dieticians to Common Nutrition Questions. J Nutr Metab. 2023;2023:5548684. Available at: https://onlinelibrary.wiley.com/doi/10.1155/2023/5548684
(5) Liao LL, Chang LC, Lai IJ. Assessing the Quality of ChatGPT's Dietary Advice for College Students from Dietitians' Perspectives. Nutrients. 2024;16(12):1939. Available at: https://www.mdpi.com/2072-6643/16/12/1939
(6) Ponzo V, Goitre I, Favaro E, Merlo FD, Mancino MV, Riso S, Bo S. Is ChatGPT an Effective Tool for Providing Dietary Advice? Nutrients. 2024;16(4):469. Available at: https://www.mdpi.com/2072-6643/16/4/469
(7) Niszczota P, Rybicka I. The credibility of dietary advice formulated by ChatGPT: Robo-diets for people with food allergies. Nutrition. 2023;112:112076. Available at: https://www.sciencedirect.com/science/article/pii/S0899900723001053
(8) Hieronimus B, Hammann S, Podszun MC. Can the AI tools ChatGPT and Bard generate energy, macro- and micro-nutrient sufficient meal plans for different dietary patterns? Nutr Res. 2024;128:105–14. Available at: https://www.sciencedirect.com/science/article/pii/S0271531724000915
(9) Hoang YN, Chen YL, Ho DKN, Chiu WC, Cheah KJ, Mayasari NR, Chang JS. Consistency and Accuracy of Artificial Intelligence for Providing Nutritional Information. JAMA Netw Open. 2023;6(12):e2350367. Available at: https://jamanetwork.com/journals/jamanetworkopen/fullarticle/2813295
(10) Papastratis I, Konstantinidis D, Daras P, Dimitropoulos K. AI nutrition recommendation using a deep generative model and ChatGPT. Sci Rep. 2024;14(1):14620. Available at: https://www.nature.com/articles/s41598-024-65438-x
Technical Terms
Systematic review: A systematic review is a comprehensive summary of all the relevant research on a specific topic. It includes multiple studies to provide a clear picture of what the science says about that topic. Studies are included such that they meet certain eligibility criteria, which are defined by the researchers. For example, the researchers may only want to look at a certain age group of people, or a certain study type. This helps to narrow the focus of the review to a particular research question.
Statistical significance: This is a term to describe the likelihood of whether a finding in a study is a ‘real’ finding, or if it is the result of chance. Statistical significance is denoted by a p-value, which is usually set at a significance (alpha) level of 0.05. This means that if a result is significant at this level (p≤0.05), we can say that the probability of getting a value as or more extreme than the observed value (under the assumption that the null hypothesis is true) is less than 5%. In other words, it is more likely that this finding is not the result of chance than if the p-value was >0.05 (although this is not always the case).